Modern Techniques And Python Tools To Detect And Remove Dirty Data And Extract

In the realm of data analysis, the quality of your data holds immense significance. Dirty data – data that is corrupted, incomplete, or inconsistent – can lead to inaccurate and misleading results, hindering your ability to make informed decisions. To overcome this challenge, data cleaning has emerged as a crucial step in the data analysis process.
The Imperative of Data Cleaning
Dirty data can manifest in various forms, ranging from missing values to incorrect data formats. Its presence can jeopardize the accuracy and reliability of your analysis, resulting in erroneous s and potentially flawed decision-making.
4.7 out of 5
Language | : | English |
File size | : | 3273 KB |
Text-to-Speech | : | Enabled |
Screen Reader | : | Supported |
Enhanced typesetting | : | Enabled |
Print length | : | 436 pages |
X-Ray for textbooks | : | Enabled |
By investing time and effort in data cleaning, you can ensure the integrity of your data and derive meaningful insights from your analysis. Moreover, data cleaning helps you:
- Identify and correct errors and inconsistencies
- Standardize data formats for seamless integration
- Handle missing values with appropriate techniques
- Enhance data quality for accurate analysis and decision-making
Unveiling the Arsenal of Modern Data Cleaning Techniques
The advent of modern data cleaning techniques and Python tools has revolutionized the data cleaning process. These powerful tools empower you to tackle even the most complex data issues with ease and efficiency.
Some of the widely used data cleaning techniques include:
- Data validation: Checking data against predefined rules to identify anomalies and errors
- Data imputation: Filling in missing values using statistical techniques or domain knowledge
- Data transformation: Converting data into compatible formats for seamless analysis
- Data standardization: Ensuring data consistency by removing duplicates and normalizing values
Harnessing the Power of Python for Data Cleaning
Python, a versatile and widely used programming language, offers a comprehensive suite of tools and libraries specifically designed for data cleaning tasks. By leveraging these tools, you can automate repetitive tasks, enhance efficiency, and ensure data integrity.
Some of the essential Python libraries for data cleaning include:
- Pandas: A powerful library for data manipulation and analysis
- NumPy: A library for scientific computing and numerical operations
- Scikit-learn: A library for machine learning and data preprocessing
- OpenRefine: A user-friendly desktop application for interactive data cleaning
A Step-by-Step Guide to Data Cleaning with Python
To embark on your data cleaning journey with Python, follow these comprehensive steps:
1. Data Loading and Exploration
Begin by loading your data into a Python environment using Pandas. Explore the data to understand its structure, identify potential issues, and determine the necessary cleaning steps.
2. Data Validation and Error Detection
Implement data validation techniques to identify errors, missing values, and inconsistencies. Use functions like isnull() and unique() to check for missing values and duplicate entries.
3. Data Imputation
Handle missing values using appropriate imputation techniques. For numerical data, consider using the mean, median, or mode as replacement values. For categorical data, use the most frequent value or create a new category for missing data.
4. Data Transformation and Standardization
Convert data into consistent formats for seamless analysis. Use functions like astype() and normalize() to change data types and normalize values. Consider creating dummy variables for categorical data.
5. Data Visualization and Verification
Visualize your cleaned data using charts and graphs to identify any remaining anomalies or errors. Manually verify the cleaned data to ensure its accuracy and completeness.
Case Study: Cleaning Real-World Data with Python
To illustrate the practical application of data cleaning techniques, let's delve into a real-world case study.
Dataset: Customer Transaction Data
We have a dataset containing customer transaction data with attributes such as customer ID, product ID, transaction date, and Free Download amount. The goal is to clean the data and prepare it for analysis to identify customer spending patterns.
Data Cleaning Steps
- Data Loading: Load the data into a Pandas DataFrame
- Data Validation: Check for missing values, duplicate entries, and invalid data types
- Data Imputation: Impute missing values for Free Download amount using the median
- Data Transformation: Convert transaction date to datetime format and create dummy variables for product ID
- Data Visualization: Visualize the cleaned data to identify any remaining issues
Results
After implementing these data cleaning steps, we obtain a clean and consistent dataset ready for analysis. We can now explore customer spending patterns, identify high-value customers, and make informed decisions to optimize our marketing strategies.
: Embracing Clean Data for Data-Driven Success
In today's data-driven landscape, the significance of clean data cannot be overstated. By embracing modern data cleaning techniques and leveraging the power of Python tools, you can transform dirty data into a valuable asset.
Investing in data cleaning pays dividends in the form of accurate and reliable analysis, empowering you to make data-driven decisions with confidence. Unlock the true potential of your data and embark on the path to data-driven success.
4.7 out of 5
Language | : | English |
File size | : | 3273 KB |
Text-to-Speech | : | Enabled |
Screen Reader | : | Supported |
Enhanced typesetting | : | Enabled |
Print length | : | 436 pages |
X-Ray for textbooks | : | Enabled |
Do you want to contribute by writing guest posts on this blog?
Please contact us and send us a resume of previous articles that you have written.
Book
Novel
Page
Chapter
Text
Story
Genre
Reader
Library
Paperback
E-book
Magazine
Newspaper
Paragraph
Sentence
Bookmark
Shelf
Glossary
Bibliography
Foreword
Preface
Synopsis
Annotation
Footnote
Manuscript
Scroll
Codex
Tome
Bestseller
Classics
Library card
Narrative
Biography
Autobiography
Memoir
Reference
Encyclopedia
Gary Mayes
Joseph Birchall
Felix Bachmann
Tyler Simmons
Rachel Gurevich
Kiara Bickers
Robert C Ankony
John Shewey
Frances Thomas
Seth Shulman
Ethan M Rasiel
Vivian Walsh
Suhas Mantri
Lorna Luft
Lia Simone Cornella
Sarah Sutton
Michael Kaehn
Felipe M S Silva
Jim Camp
Robert D Novak
Light bulbAdvertise smarter! Our strategic ad space ensures maximum exposure. Reserve your spot today!
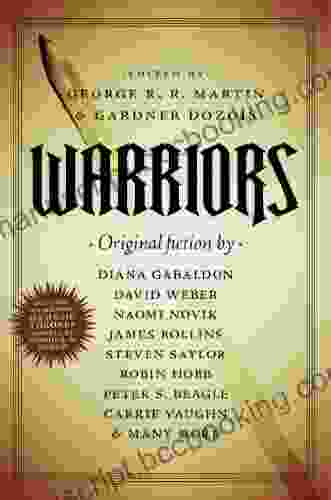

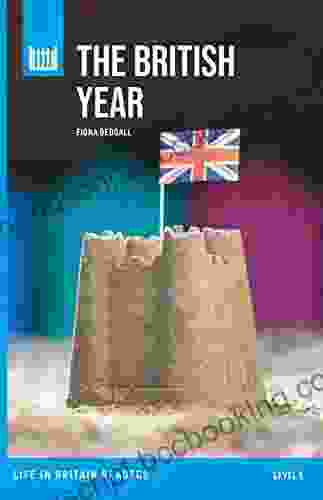

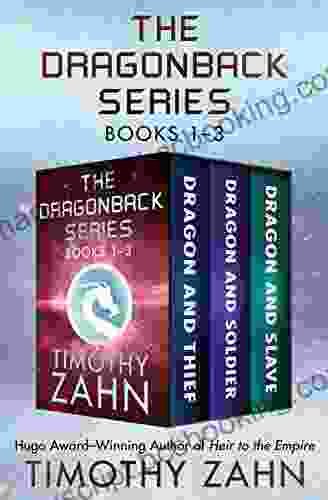

- Morris CarterFollow ·5.8k
- Greg CoxFollow ·16.1k
- Manuel ButlerFollow ·19.6k
- Bryce FosterFollow ·4.6k
- John GreenFollow ·6.7k
- Todd TurnerFollow ·11.5k
- Edmund HayesFollow ·15.2k
- Italo CalvinoFollow ·17.4k
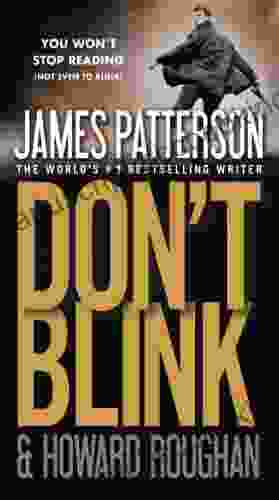

Step into a World of Thrilling Deception: Don Blink by...
Unveiling the Masterpiece of Suspense:...
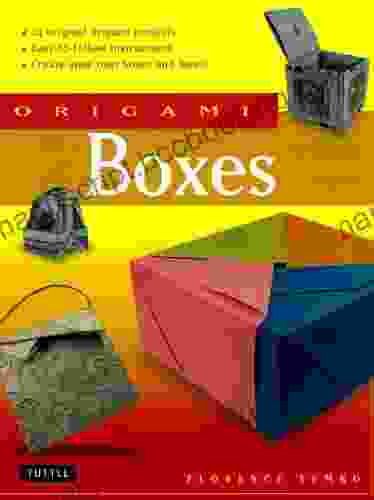

Unleash Your Creativity with "This Easy Origami": A...
: Embark on an Enchanting Voyage into the...
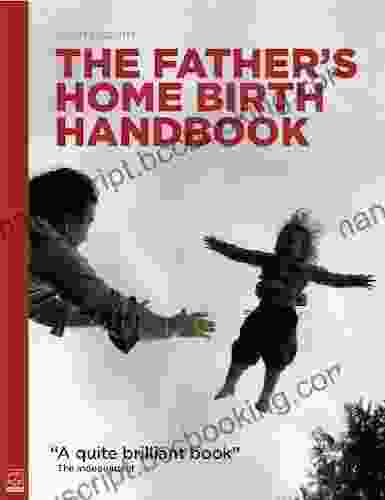

Empowering Home Births: A Comprehensive Guide for Fathers...
An In-Depth Exploration of Paternal...
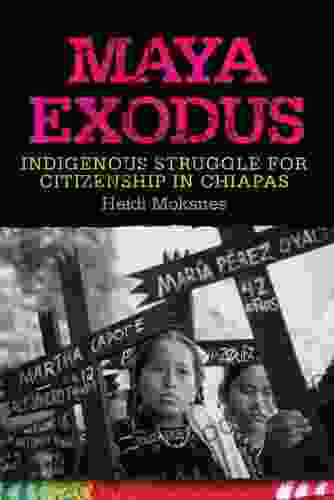

The Maya Exodus: Indigenous Struggle for Citizenship in...
The Maya Exodus: Indigenous Struggle for...
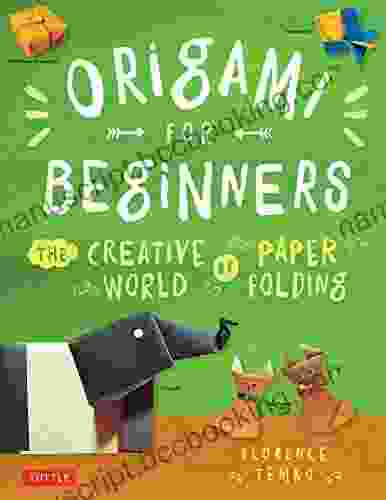

Kana Made Easy: Dive into Japanese the Fun and Effortless...
Unveiling the Secrets...
4.7 out of 5
Language | : | English |
File size | : | 3273 KB |
Text-to-Speech | : | Enabled |
Screen Reader | : | Supported |
Enhanced typesetting | : | Enabled |
Print length | : | 436 pages |
X-Ray for textbooks | : | Enabled |